DS2 White Papers
The SHARPS DS2 team has authored the following companion papers, Technical and Architectural Considerations and Contextual Integrity Considerations:
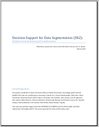 | Decision Support for Data Segmentation (DS2): Technical and Architectural Considerations  Abstract: In response to demand for solutions to implement privacy protections for certain types of electronic healthcare data while allowing sensitive health information to flow to authorized users, the health information technology community has been developing technical standards and solutions in a topic area known as Data Segmentation for Privacy, or DS4P. This paper presents the results of a research-oriented project to demonstrate that certain DS4P tasks can be enhanced through the use of clinical decision support (CDS) technology. It advances a novel use of CDS tools to 1) identify and sequester certain types of information from electronic medical records and to 2) help mitigate the potential risks of exchanging records from which data have been sequestered. The approach is called Decision Support for Data Segmentation, or DS2. It builds upon standards-based open source CDS technology to create a familiar CDS-based platform for the development and testing of functions to identify and redact selected conditions from clinical summary documents in various contexts including Health Information Exchange (HIE) between healthcare providers. The DS2 prototype demonstrates how deterministic clinical rules and machine learning-based classifiers can work together to detect clinical facts that may imply a condition even if they are not directly related to the condition and how CDS at the point-of-care can potentially make use of clinical information even after it has been sequestered.
|
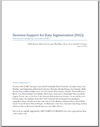 | Decision Support for Data Segmentation (DS2): Contextual Integrity Considerations  Abstract: Data segmentation is a concept that describes the process of sequestering elements in electronic records that are perceived as being undesirable to share in a particular context. In the face of increasingly ubiquitous flows of electronic health information, policy makers have called for the development of technologies that would enable data segmentation. Viewed as a means of giving patients more granular control over flows of their health information, data segmentation is thus a potential privacy-protecting strategy. Indeed, research into patient views of health information privacy and electronic health information flows suggests that patients want the capacity to segment their data. However, as data segmentation technologies are still nascent, there are important limitations associated with deploying data segmentation as a privacy-protection strategy. Chief among these limitations is that informed observers may be able to infer information that patients do not want to share, even after that information has been sequestered (we refer to this as the inferencing problem). This White Paper proposes some novel approaches for addressing the inferencing problem. In conversation with the federally-sponsored “Data Segmentation For Privacy” (DS4P) initiative, it first discusses key challenges of data segmentation. It uses Helen Nissenbaum’s Contextual Integrity framework to argue for the development of a more context-aware definition of data segmentation. It also discusses a research-oriented project demonstrating that certain DS4P tasks can be made more context-aware through the use of clinical decision support (CDS) technology. Dubbed Decision Support for Data Segmentation (DS2), this project advanced a unique use of CDS tools to (1) identify and sequester certain types of information in electronic medical records and to (2) mitigate potential risks of exchanging records from which data have been sequestered. It contributed a variety of tools that could be leveraged by existing DS4P pilot projects, including a new technical architecture and prototype, a suite of related open-source software tools, and test data for evaluating the application of various machine learning techniques to address the inferencing problem. Our emphasis on contextual integrity considerations, and our engagement with the clinical inferencing problem, illustrate DS2’s potential to help health information stewards and patients better understand the complex, context-aware behaviours of segmented records and corresponding data segmentation strategies.
|
Other DS2 Publications
Decision Support for Data Segmentation (DS2): Application to Pull Architectures for HIE 
Carl A. Gunter, Mike Berry, and Martin French. USENIX Summit on Health Information Technologies (HealthTech ’14), August 2014. Presentation video available at USENIX website.
Other Publications by Members of the SHARPS Team
Understanding the Challenges with Medical Data Segmentation for Privacy 
Ellick M. Chan, Peifung E. Lam, and John C. Mitchell. USENIX Workshop on Health Information Technologies (HealthTech ’13), August 2013.
Visit http://sharps.org/publications for a complete list of SHARPS publications.